Intel 4th Gen Xeon Scalable Sapphire Rapids Performance Review
Intel 4th Gen Xeon Scalable CPU: Accelerated Workloads And Conclusion
SMHasher 1.1.0 Security Processing Benchmark
SMHasher is a benchmark tool to assess the performance of various hashing algorithms. Such workloads pop up all over in the datacenter and are often critical for security applications. Wyhash is not considered completely secure, but it is very efficient, portable, and fast.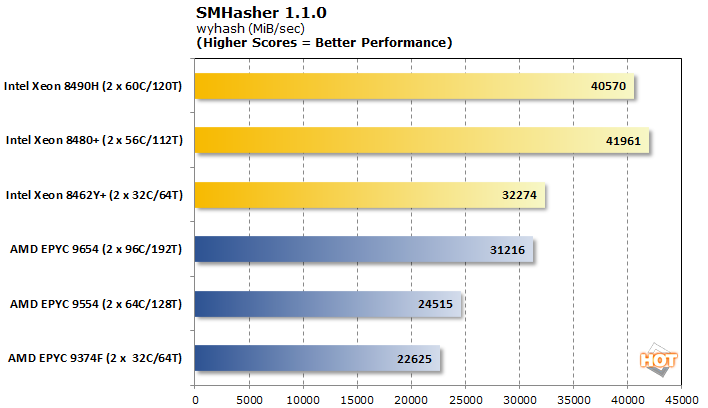
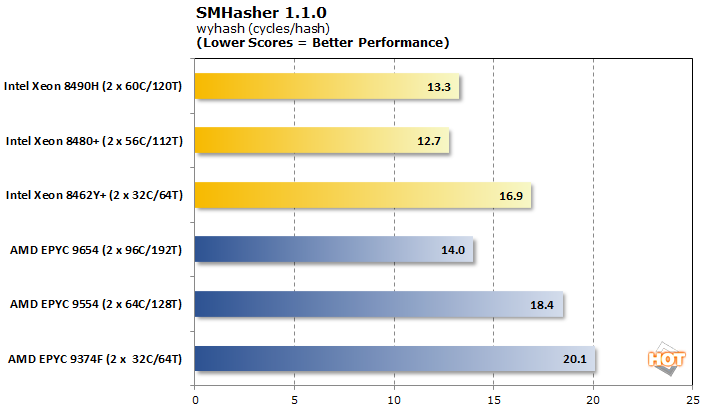
With AVX in full swing, the Intel trio puts up some impressive numbers. Even the Xeon 8462Y+ beats out the EPYC 9654 with three times its core count in throughput, though the latter does burn fewer cycles per hash.
Intel Open Image Denoise 1.4.0 Benchmark
An important aspect of ray tracing is the denoising step which cleans up the image for a better-looking frame. Part of oneAPI, Intel Open Image Denoise is one such library to accomplish this which can be used not only for gaming, but also for animated features.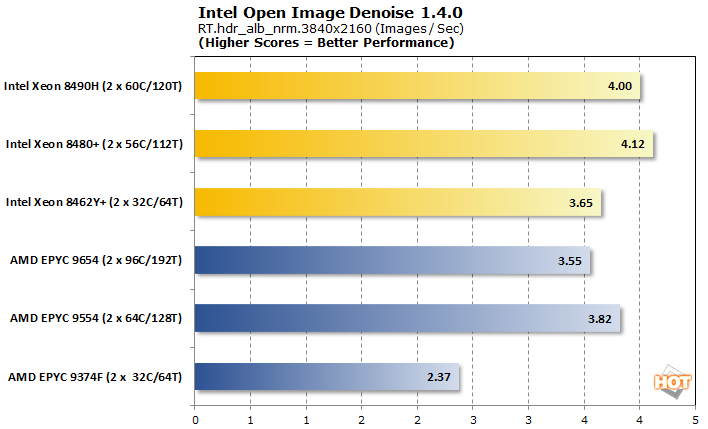
This is another close comparison, with five of the six test CPUs putting up strong results. The scales tip somewhat in favor of Intel, as the accelerators allow even the Intel Xeon 8462Y+ to keep pace with the pack while the EPYC 9374F falls off considerably.
TensorFlow 2.0.0 Machine Learning Image Classification Benchmark
TensorFlow offers a few different models to analyze with, so we tested VGG-16, AlexNet, GoogLeNet, and ResNet-50 for pre-trained neural network image classification workloads.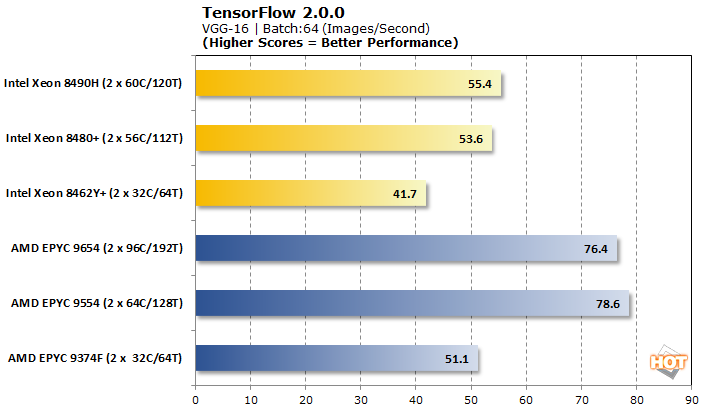
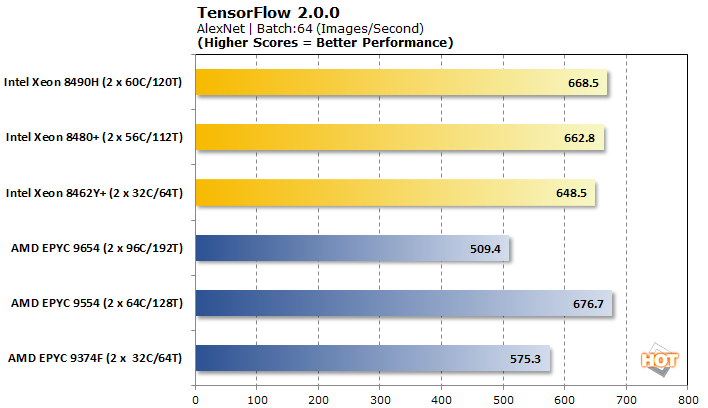
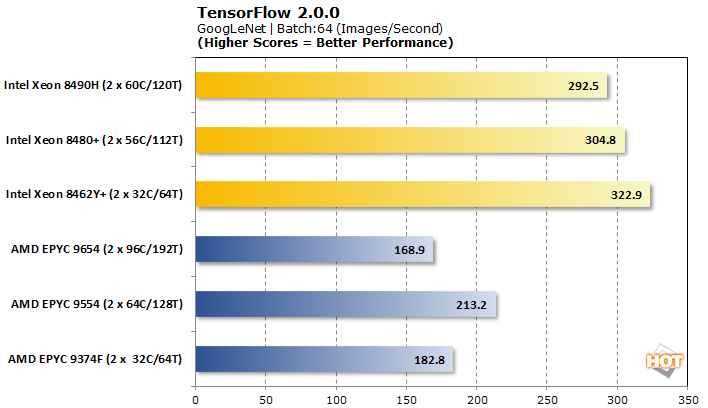
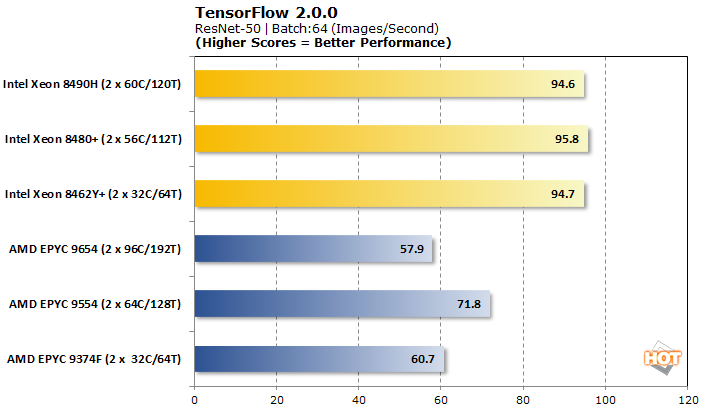
Tensorflow is another benchmark where AMD EPYC Genoa processors have generally picked up ground since launch. Even still, Intel lands pretty decisive victories in three out of the four workloads. Only VGG-16 is better-served on some AMD processors. Performance scaling within a brand is a bit all over the place, ranging from nearly non-existent on Intel to downright erratic for AMD.
DeepSparse AI Inferencing 1.0.1 Benchmark
Neural Magic’s DeepSparse differs from many AI inferencing approaches, in that it is developed specifically for CPUs. As the name implies, the models are pruned extensively (e.g. sparsified) to deliver “GPU-Class” deep learning on CPUs while retaining a high degree of accuracy.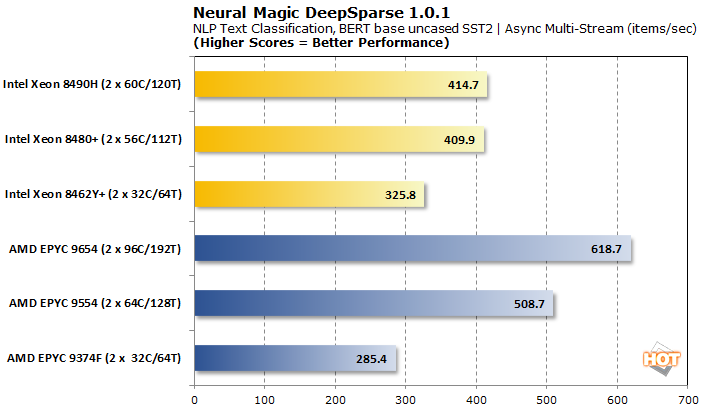
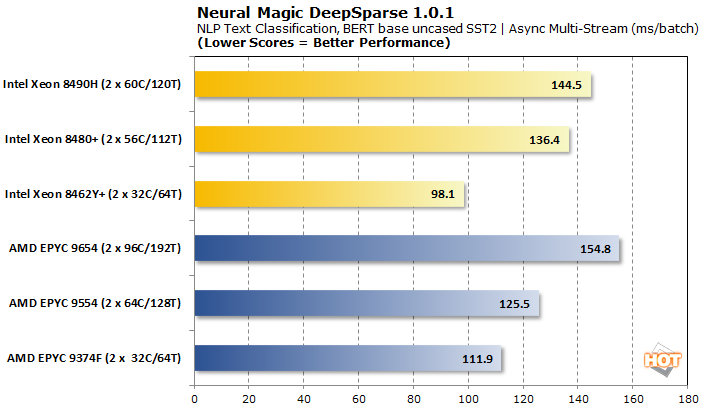
With asynchronous multi-stream batching, the Intel Xeons retain competitive per-batch latencies to AMD EPYC. The resulting throughput is quite a bit lower, though, 32-core parts aside.
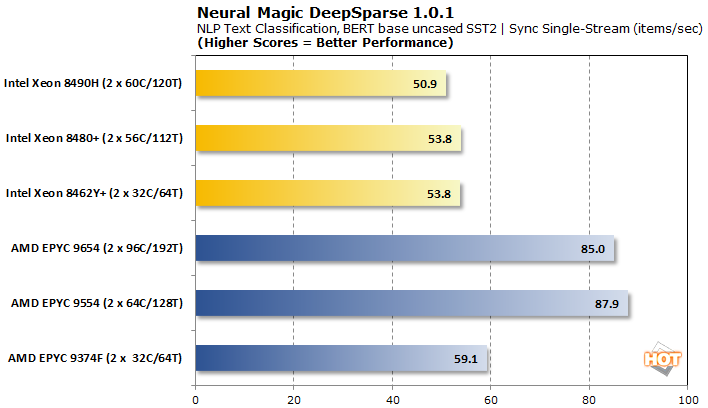
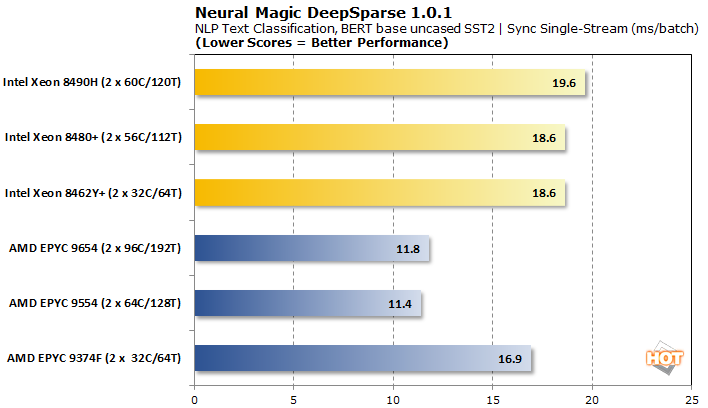
Moving to a synchronous stream, the AMD EPYC CPUs pull solidly in front with lower latencies as well. The EPYC 9374F lags a bit in both respects, but still beats out all Xeon contenders.
OneDNN 3.0.0 RNN Training And Inferencing Benchmark
OneDNN is an Intel-optimized open-source neural network library that is now part of oneAPI. Our testing looks at its performance in convolution, RNN training, and RNN inferencing across three data types: f32, u8u8f32, and bf16bf16bf16.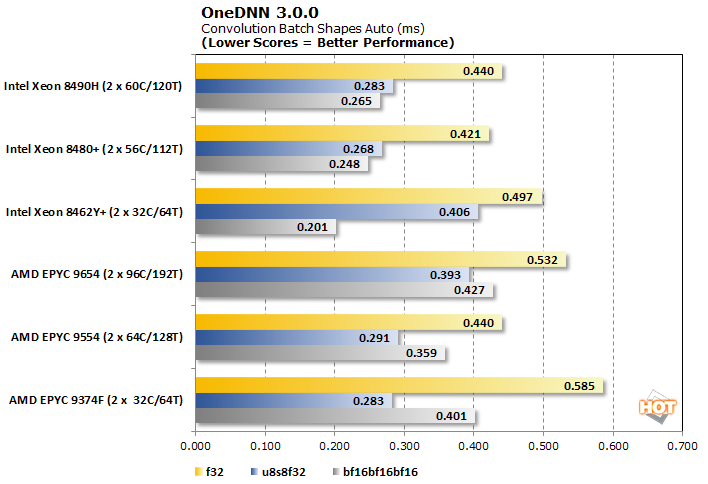
These results vary from a mild advantage for Intel Xeon CPUs with f32, to a rather smashing victory with bfloat16. Curiously, the u8 (unsigned 8-bit) workload favors the higher core-count Intel SKUs, but instead the lower core count chips in AMD's Genoa family.
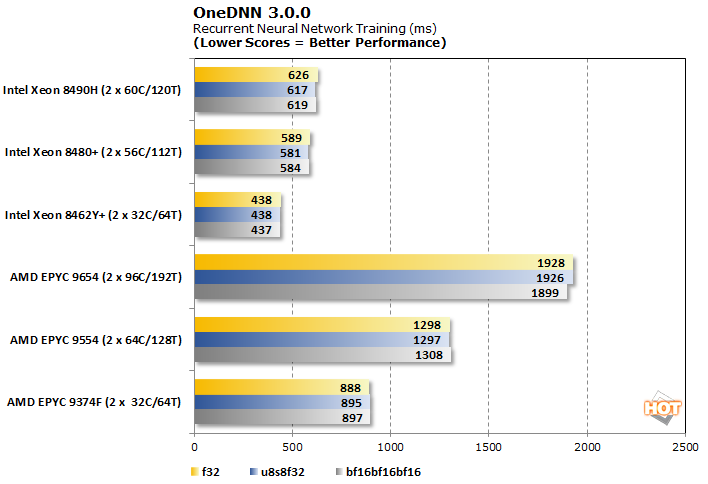
The recurrent neural network training workload is virtual data type agnostic for all SKUs. We can observe a preference for lower core count systems to achieve better latency, but the Intel Xeons overall are far and away faster in this scenario.
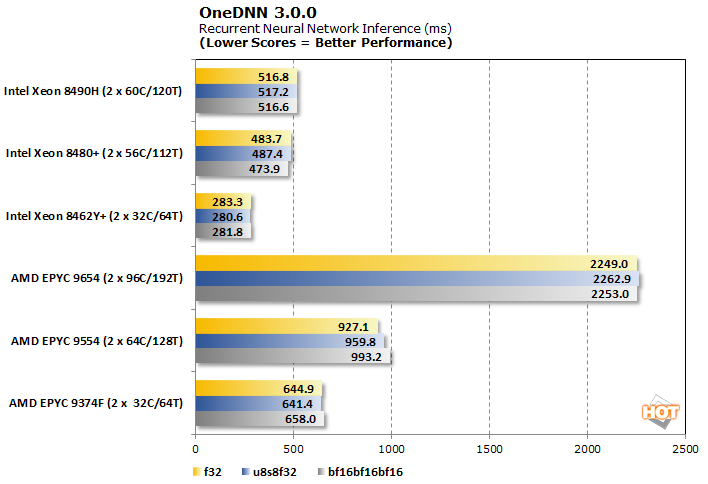
The inferencing component also shows an Intel landslide victory, and the AMD EPYC 9654 particularly lags here despite its 96 cores of brute force power.
Intel 4th Gen Xeon Scalable Final Thoughts And Conclusions
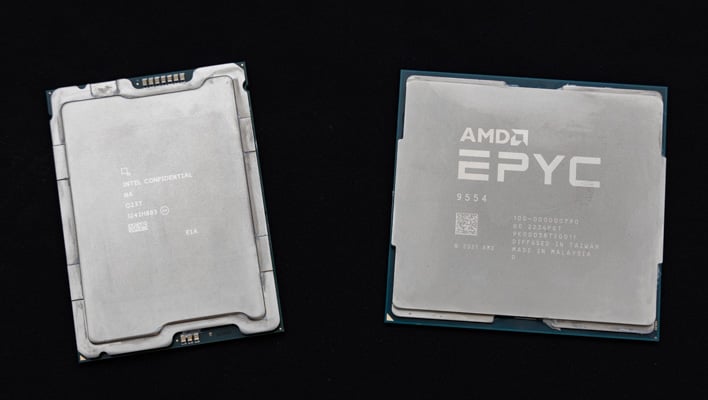
Evaluating Intel’s 4th Gen Xeon Scalable processors is certainly tricky business. For conventional workloads, AMD’s EPYC processors are proving very difficult to beat. Even if we remove the 96 core EPYC 9654 from the equation, AMD seems to deliver more on a pound-for-pound (core-for-core) basis.
General purpose compute is far from the full story in the modern data center, though. Developers are tapping into neural network applications more and more to tackle big data analytics problems and uncover new solutions. While most of this work is taking place on GPUs and dedicated accelerators (e.g. FPGAs), Intel is proving that a CPU can get the job done too with potentially lower cost and overall footprint. As a result, it can make sense to grab one of these 4th Gen Xeon processors where the accelerators can be leveraged, like reaching for the right tool for the job.
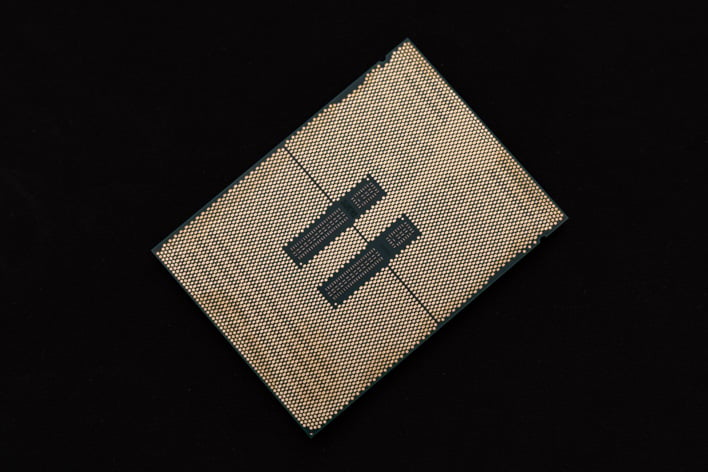
Our testing here does not completely explore mixed workload scenarios as well. A key advantage of the discrete accelerators is that the CPU cores are effectively freed up for other jobs. As a result, real-world applications should be able to leverage both simultaneously (power and thermals permitting), with different concurrent tasks for an even greater effect.
We're hoping to return to this evaluation in the future, with combination workloads that leverage both the on-board accelerators of Intel's 4th Gen Xeon family, along with general purpose compute workloads, concurrently.
Regardless, the formula is more complicated than “more cores = better” for Intel, though. The discrete nature of the accelerators can mean that customers shopping for the capabilities of that accelerator may find they can disregard some of the remainder of the CPU spec sheet. Lower core count Xeons can yield lower latencies and better per-core clock speeds, often giving them the edge in AI type workloads, for example.
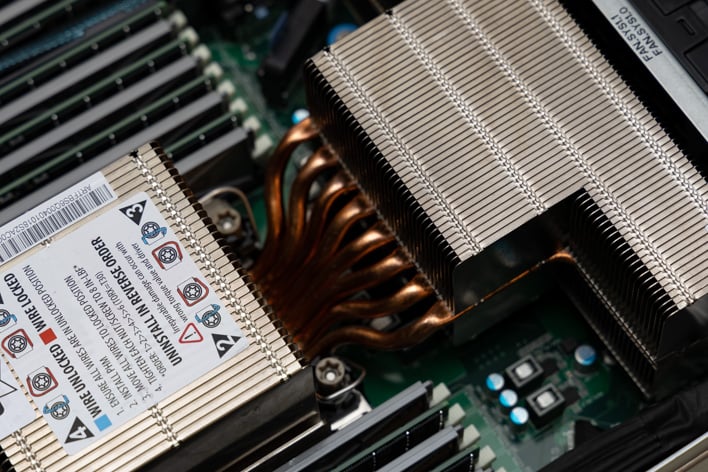
The other elephant in the room is Intel’s “On Demand” service model. Apart from the “fully enabled” -H series and HBM-equipped Xeon Max products, customers who want the accelerators (or more of them) enabled may be faced with an extra bill to license them. Sure, this can arguably give other customers a lower cost of entry, but those same customers may be better suited in the AMD camp anyway. It also does not change the fact that the accelerators exist fully functional in silicon, they just cannot be used without a fee.
We also want to point out that Intel’s accelerators are not limited to just AI type workloads. Intel has accelerators for networking, dynamic load balancing, data streaming, in-memory analytics, and more. Based on what we have seen, we think we absolutely can expect to see a wider variety of purpose-built silicon moving forward. Intel’s tiled chiplet approach could also open the door for some truly custom recipes for individual customer demands.
